Real-time project and real-life experience
When building a house, it is crucial to know whether the ground consists of rock, gravel, sand, or clay. The same goes for underwater installations, but it is not so easy to determine as on dry land.
CIUS partner Kongsberg Maritime wished to explore whether deep learning could assist in real-time seabed classification, and our PhD Candidate Rosa Virginia Garone stepped up to the challenge.

“The knowledge helps the scientific community to make important decisions about the management of marine resources, ecosystem preservation, offshore installations (both for the oil & gas and the renewable energy industries), offshore structural monitoring and hazard control,” Garone explains about the motivation for the project.
Seabed sediment classification is usually a manual process involving expert operators merging geological, geophysical, and geotechnical data to infer information about the distribution of the sediments. This can be very time-consuming and subjective.
“With the use of deep learning we aim to automate or partially automate the process of sediment mapping by letting the neural network predict the sediment classes, thus reducing the subjectivity and increasing speed. So far, we hadn’t the opportunity to conduct these experiments in real-time” Garone says.
A real(-time) problem
To investigate the potentials of a deep learning network to solve a seabed classification problem in a real-time setting, Kongsberg Maritime announced a summer project where Garone and other students with different technical backgrounds were chosen to work on these problems. Garone’s background made her particularly qualified for this.
She has a bachelor in geology and a master’s degree in geophysics, and has experience as a geophysicist/geologist and processing geophysicist before she started her PhD in CIUS. Her PhD project uses Deep Learning for seabed sediments classification and is the result of a collaboration between CIUS, Kongsberg Maritime and The Geological Survey of Norway (NGU).
She heard about the summer project via her co-supervisor at Kongsberg Maritime Tor Inge Birkenes Lønmo. The two-month summer project spanned across different topics and different engineering disciplines and explored a practical application of deep learning for seabed sediments classification. The Deep Learning models tested during the Summer project were trained with the MBES data and geological maps provided by NGU.
“I was immediately thrilled about it and interested in answering two main questions. 1) Is my algorithm able to generalise its predictions in a different geological setting without any need for re-training the neural network? And 2) What about real-time context? What are the factors affecting the quality of sediment class prediction then?”
For her initial experiment, Garone used deep learning to discriminate between hard substrate (mainly bedrock) and soft sediments (mud or sand or mixed sediments). To do this, the neural network has been shown expert interpretation of the bathymetry and backscatter so that it can learn to predict and discriminate different sediment classes.
“My main task consisted in adapting my PhD work to MBES data from a different geological background and geographical location. Thus pre-processing and processing of the new data, together with the deep learning algorithm integration into the real-time pipeline had a huge impact on the positive outcome of the experiment,” Garone says.
“Results looked encouraging despite the biggest challenge was related to obtaining MBES data without artifacts and with a low signal to noise ratio,” she adds. “In fact real-time data don’t undergo all the necessary pre-processing steps necessary to make them ideally clean from artifacts and noise”.
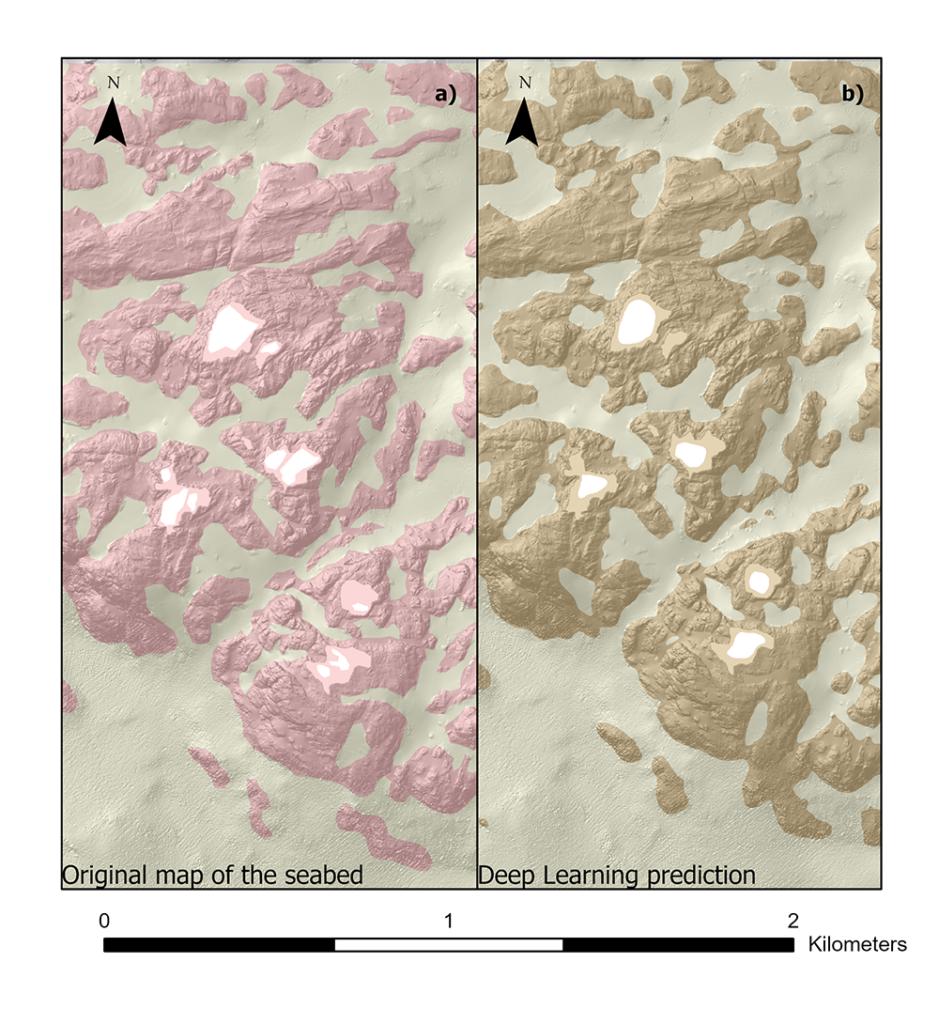
A step towards automated classification
Obtaining high quality MBES data is not just a question of equipment. External factors also play a major role: Ocean depth and weather conditions affect the quality of the MBES data and images, which again affect the performance of the deep learning algorithms:
“Ultimately our final results looked encouraging. The algorithm provided a plausible map of the seabed sediments. However, due to the scarcity of good quality images and missing ground truth we were not able to do a rigorous comparison.”
“It is very encouraging to see that Rosa and the students were able to integrate the algorithm in real time during this summer project. Something like this would be very valuable for many users of multibeam systems, and this shows that a real time implementation could be feasible,” comments Birkenes Lønmo.
Real-life experience
The summer project had more than just scientific benefits:
“This collaboration gave me the opportunity to step out of my comfort-PhD-project-zone, challenging me to find a solution to a real-time, real case problem,” Garone reflects.
“It was extremely interesting to come out with different solutions to different problems together with the other students participating in the summer project. This experience has benefitted me academically by pushing me to keep in mind how important it is being able to make the results of research available for use in a real-case scenario. This will for sure also help me careerwise,” she concludes.
CIUS would like to add a thank-you to NGU for contributing to this project and for providing the data used.
Multibeam Echo Sounder (MBES)
Multibeam echo sounder is type of sonar system used to map the seabed. These instruments have the capability to map the seafloor by emitting narrow acoustic beams allowing for a 100% coverage of the ocean bottom.
Backscatter Mosaic
The backscatter mosaic is a product of the MBES systems. Backscatter data provide the users with a reflectivity seabed map. In fact, different seabed sediments have distinct acoustic characteristics, as a consequence, the acoustic wave emitted form the MBES instrument will be reflected from the seabed accordingly to the seafloor sediments characteristics.
Bathymetry Grid
The bathymetry grid is an additional product of the MBES systems. Bathymetry data provide the users with information about the seafloor depth and its spatial variability. Depth data are acquired by measuring the time necessary to the MBES instrument’s acoustic wave to hit the seafloor and to be reflected back to it.
This article first appeared in the 2022 CIUS Annual Report.
-
Kari Williamsonhttps://www.ntnu.no/blogger/cius/author/kari_williamson/
-
Kari Williamsonhttps://www.ntnu.no/blogger/cius/author/kari_williamson/
-
Kari Williamsonhttps://www.ntnu.no/blogger/cius/author/kari_williamson/
-
Kari Williamsonhttps://www.ntnu.no/blogger/cius/author/kari_williamson/