Challenges and potentials of visual computational analysis Insights from a study on politicians’ self-depiction and their news portrayal
Visuals are omnipresent in contemporary online political communication. This can be considered a consequence of the development of new and more visual social media platforms such as Instagram, and has been fueled by the increasing mediatization and personalization of political processes. Research from psychology indicates that visuals have the potential to affect recipients’ political opinion at an early stage and on a fundamental level (see Alexander Todorov and colleague’s study in Science). As a result, politicians have increasingly professionalized their visual communication efforts. Simultaneously, social scientists are increasingly interested in analyzing the role of visuals in contemporary political communication (see the post by Uta Russmann on this blog). In this, new opportunities to download and analyze large corpora of visual data offer new insights into visual political communication.
The outline of the study
In a recently published study, we conducted a visual computational analysis to compare the visuals political candidates distribute on their Twitter and Instagram accounts with the visuals of the same candidates that are distributed by news media. In the following, we use this study as an example to demonstrate how such a research endeavor can be conducted and what challenges and potentials are offered by visual computational analysis.
The gist of the study is that politicians are eager to distribute visuals that display them in a favorable way, while mass-media logic focuses more on negativity and conflict. But what are favorable images? In the process of identifying indicators of favorability that we are also able to measure computationally, we turned to studies on the effect of visuals (for instance this study by Katharina Lobinger and Cornelia Brantner). Here, we identified the following potential differences in the favorability of visuals (1) the depiction of politicians as happy, (2) the use a worm-eye camera angle, (3) the display of more than one person and (4) the use of close-up perspective.
The Methodological Procedure
We compared the visuals distributed by candidates via Instagram and Twitter during the 2019 European Parliamentary Election to the visuals published in online news media. Therefore, we had to compile a list of all official candidates. For each party there were lists of candidates available online in differing quality ranging from ready-to-use CSV-files to scanned PDF documents, sometimes even corrected in handwriting. As a result, creating the final list of 13,811 names was a labor-intensive endeavor that required the help of colleagues with various language skills to get the downloaded lists into machine-readable formatting.
Next, we matched the candidate names to their Twitter and Instagram accounts. For Twitter, we relied on the Twitter API to search for each candidates’ full name and selected – if available – the top-most verified profile among the first five search results. Alternatively, we selected the top-most non-verified account from the first search result. Through this procedure, we were able to identify 7,588 Twitter profiles (55% of all candidates), 711 of which (9%) were verified. Since Instagram does not provide a search function as part of their API, we conducted the respective searches through the platform’s desktop website using common scraping tools. This helped us to identify 8,530 Instagram profiles (62% of all candidates), of which 291 profiles (3%) were verified.
To ensure that the identified accounts belong to the respective politicians, we drew a random sample of 150 candidates and looked at the identified profiles. For Twitter, 68 out of 84 non-empty profiles (81%) were attributed correctly, a rate usually deemed accurate. For Instagram, however, only 51 non-empty profiles (61%) were attributed correctly. This level of accuracy would usually not be considered adequate. However, given that the electorate can be expected to similarly search for full names, also relying only on search results, we decided to continue with our data collection.
We then collected imagery for all candidates from their Instagram, their Twitter, and the two most-read news outlets in each EU country. For Twitter we scraped the profile picture as well as the profile’s banner. From Instagram we scraped the profile picture as well as the last five posts. Finally, for the media coverage, we adopted a script by Peng (2018) which we used to search on Bing for images on the news outlets’ URL that included the candidates’ name and scraped the latest five search result. In total, this resulted in 79,500 images. All data and scripts are available online.
We used the popular computational vision service Face++ to code the visuals. For every single visual uploaded through the service’s API, Face++ responds with a list of identified faces, including (but not limited to) the number of depicted faces, a face’s position and size, its pitch angle (from −180° to +180°), and the likelihood of a face being happy (a percentage). For our analysis, we re-coded pitch angle into three groups, namely worm-eye, bird-eye, and straight perspective. Per image, the dominating group of face angles then determined the image’s perspective. Similarly, a picture was considered happy if at least half of all depicted faces were happy. We also re-coded the accumulated surface area for all faces in relation to an image’s size into whether a picture employed a close-up proximity.
To ensure validity of these measures, we manually coded 300 visuals. The agreement between both coders was acceptable for all variables. The reliability between the manual coding and the computational results was acceptable for the number of faces and happy facial expression, while it was below acceptable thresholds for the camera angle. Consequently, results about the applied camera angles had to be treated with caution.
The main takeaways
Our findings show that politicians more often than the news media distribute pictures containing happy facial expressions – although the degree of happiness varies systematically across the different EU countries. The number of depicted people does not vary between news media and social media, since most images depict either one or no person at all. Finally, news depiction employs significantly more bird-eye and worm-eye perspectives as compared to self-depiction. Overall, the results suggest that even though some aspects of favorability are more clearly pronounced in politicians’ self-depictions, others are not. These differences are largely independent form the party family a politician belongs to, while the country in which a politician runs for election is a consistent yet minor predictor for all measured variables.
Main Methodological Take-Aways
Using Face++, a third-party provider to code images, is partly intransparent. This can be problematic as it potentially affects privacy concerns, impedes replication, and is vulnerable to systematic bias. Face++, for instance, might update its trained models, which would impede replication as a repeated coding of the same material might lead to slightly different outcomes. Similarly, while our analysis is based on a wide variety of European candidates, automated classifiers have been shown to create results of different validity for people of different appearance and gender. That said, also manual content analysis cannot guarantee full validity or reliability. As such, while our validation shows highly sufficient validity scores for all but one category, it remains unclear why the remaining face-angle category lacks satisfying intercoder reliability. There are two potential explanations for this: First, there might be a lack of conceptual clarity and thus a misconception of the category itself. Alternatively, low reliability might be a consequence of a fallacy within Face++. In any case, once the validity of a computational analysis proves satisfying, our approach allows for study scales way beyond what would have been possible solely through manual content analysis. It is, however, crucial to validate coding for every conducted study with regard to every category used.
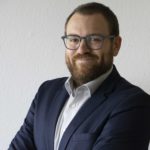
Marc Jungblut
Marc Jungblut is a postdoctoral researcher at the Department of Media and Communication at LMU Munich. His research focuses on the role of media in conflict, computational social science and strategic communication.
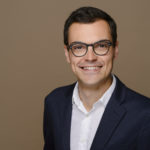
Mario Haim
Mario Haim is an Assistant Professor at the Department of Communication and Media Studies at the University of Leipzig, Germany. His research focuses on computational journalism, news use within algorithmically curated media environments, and computational social science.